March 3: D. Cale Reeves, Georgia Institute of Technology's School of Public Policy
"Generating Insight from the Bottom Up: Agent-Based Modeling of Home Energy Technology Diffusion"
View a recording of this talk.
ABOUT THE SPEAKER
cale_reeves.png
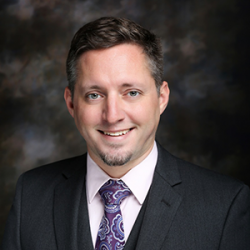
D. Cale Reeves is a Visiting Assistant Professor at Georgia Institute of Technology's School of Public Policy. He explores the individual-level decision-making processes that drive emergent policy outcomes, focusing mainly on understanding the green technology adoption/diffusion system. As a computational social scientist, he enjoys applying cutting-edge methods such as agent-based modeling and natural language processing to problems that require broad systems-aware approaches, thus contributing to the search for solutions for so-called "wicked problems" like climate change. He hopes to understand how the exchange of information within social networks can be explicitly leveraged in intervention designs to achieve more effective programs and more efficient policy.
When he is not at school, Dr. Reeves is probably either fixing a 1969 Triumph 500 or riding a 2016 Zero DSR.
ABOUT THE TALK
Modeling helps us to understand what things are like (to paraphrase Russell Ackoff). There is a vagueness in this statement that is fundamentally appealing: things can be like a lot of other things. Thus, there are many ways to model phenomena and each is capable of yielding important insights. Alongside top-down approaches that describe entire systems at a macro-level, there are bottom-up approaches – often operationalized as agent-based models (ABMs) – that treat macro-level system characteristics as emergent from micro-level behavior. Applied to the diffusion of home energy technologies (e.g. solar photovoltaics, high-efficiency HVAC, smart thermostats, etc.), ABMs can reveal novel and actionable insights that stem from a rich description of how people, embedded in complex system, approach technology adoption decision-making. In this presentation, Dr. Reeves will showcase findings from several such models, including insights into:
- disambiguating accelerated adoptions from additional adoptions
- practical tools to decrease informational barriers to adoption
- expectations of the distributional impacts – i.e. equity – of policy decisions
The presentation culminates in an exploration of how modeling home energy technology co-adoption is like modeling COVID-19, where Dr. Reeves shows how lessons learned from epidemiology can set a path forward for modeling how individuals decide to co-adopt suites of related technologies.